The global AI industry is predicted to reach $190.61 billion market value in 2025. The increasing growth in the use of AI and ML models has led to a boom in the requirement of data annotation, with an expected growth of 32.54% CAGR from 2020 to 2027. Developing an AI/ML model requires huge amounts of training data and the biggest challenge remains to get access to high-quality training datasets. Data quality is one of the reasons AI projects succeed, fail, or overshoot budgets of AI and ML companies.
The success of Artificial Intelligence (AI) and Machine Learning (ML) applications completely depends on data and data quality and that is the reason on average, 80% of the time spent on an AI project is on data labeling. You need to identify your project requirements, find out the volume of data needed, organize and clean your data, put a quality check process in place, and structure the workflow. High-quality data is a mandatory requirement for the success of AI and ML models. And it is imperative to comprehend how to gather and prepare your data for effective data labeling. Poor quality data will lead to flawed AI models.
That said, procuring high-quality data has its challenges. Multiple data quality issues affect data labeling, posing a threat to your ML/AI projects.
What affects data quality?
Before we talk about the considerations to accelerate data quality, let us dwell on what affects data quality and accuracy in data labeling for AI.
The quality of your data may drop due to challenges faced with workforce, processes and technology. If your workforce lacks domain knowledge and contextual understanding, there will be accuracy issues in labeling. Moreover, they also need to be agile, as ML is an iterative process requiring multiple tests and model validation.
The ability to respond to constantly changing workflow based on tests and validation is crucial for high-quality data labeling. And then, choosing the right data labeling tool is important to maximize quality. Finally, the dataset itself must have sufficient balance and variety for the algorithms to be able to predict similar points and patterns.
5 Important considerations to accelerate quality data labeling
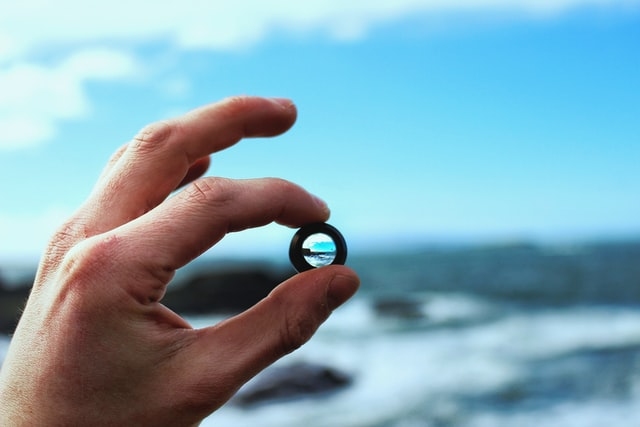
There are 5 major considerations if you are to up quality by optimizing data labeling accuracy and efficiency for AI.
1. Balance data points for algorithms to predict better
There are various types of annotations depending on the form of data, such as text, audio, video, image, etc. In fact, according to the 2020 State of AI and Machine Learning report, organizations are using 25% more data types in comparison to the previous year. Based on your business goal, identify the data that needs annotation. Keep data diversified so that you can infer ML models in multiple real-world scenarios, but again at the same time keep it specific to avoid any errors. Understand your requirement; every case requires a specific approach. If your project is on autonomous vehicle training, you should have the images of both moving cars and parked vehicles equally distributed. This will help to train AI in differentiating between moving and motionless vehicles. Also, ML being an iterative process, you will need to keep adding datasets and enriching the existing ones. Choose the data that best fits your business goal and then move ahead.
2. Optimize and ensure data quantity needed to train MLM
Once you have identified the data type, next you need to figure out data quantity. Based on project requirements, the amount of data needed can be fixed. Large quantities of quality training data help machines understand better and therefore, the more annotated data you use to train a model, the smarter it becomes. For any ML project, huge volumes of data need to be labeled, and this is where a skilled data annotator comes into play.
The size of the dataset you would need depends on the kind of results you are looking for, the complexity of the model and, even to some extent, on the time frame. You may start with simple models requiring lesser data points before you plunge into complex models requiring huge volumes of data. The more complex your models, the more data will be needed. Suppose you are writing a model to identify cars. Then you need to have thousands of images that have the label of car/non-car images.
Data benchmarks should be set after careful consideration of project goals. If you are not able to collect the amount of data required for your project, you could opt for data augmentation, data synthesis or use discriminative methods. But these methods do have their limitations and may not have the required effects if your initial dataset is of too small a size or not well distributed. In that case, you have no option but to collect new data points.
3. Data quality for the success of ML models
Data quality is one of reasons for the success or failure of AI projects, where 80% of work goes in data preparation. According to Andrew Ng, the founder of deeplearning.ai and former head of Google Brain, a lot of problems could have been sorted if more focus was shifted to improving data than code. He believes that ML projects can be accelerated if the process becomes data-centric rather than model-centric. AI being a complex technology requires properly labeled data and therefore monitoring data quality is a necessity.
Before starting any AI project, ensure your data is clean. Data cleanliness plays a vital role in labeling. Data from multiple structured and unstructured sources may have errors. Experts in the field use automated data cleansing tools and technology-enabled solutions to prepare data for training.
Once your data gets clean, take it up for labeling. Training computer vision systems with inaccurate and incomplete data can prove disastrous, especially in critical sectors like the healthcare or automobile industry. Both accuracy and consistency of the labeled data are important for data quality and it should be assessed both manually and through automation.
4. Measure training data quality through QA process
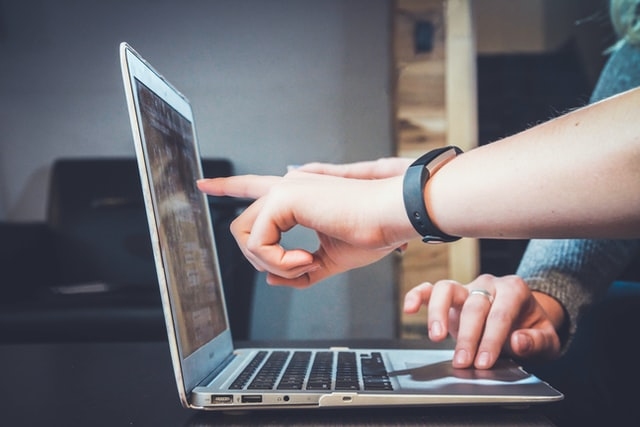
For a machine learning model to work successfully, the labels on data need to be accurate, unique and informative. QA ensures that data meets all these requirements. You can have the process in-house, automate it or even look for a good service provider offering QA service.
Integrate a QA methods into your project pipeline to assess the quality of the labels. Some of the standard quality control methods used to ensure data quality includes benchmarks (aka gold standard), consensus, and review. Benchmarks ensure accuracy by comparing the annotations to a vetted benchmark established by data scientists. This process provides a useful reference system, and you can keep measuring your output’s quality during the project. Consensus measures the consistency among the group. It works by calculating a consensus score by dividing the sum of agreeing labels by the total number of labels per asset. In auditing, the quality is reviewed by experts. A specialist can figure out which QA services will be best suited to your project requirement.
5. Choose the right approach to get annotation work done
Assignment of the labeling task is important for the success of the project, and keeping the budget in check. Data labeling for AI is a complex and time-consuming process where data collection, processing, play vital roles. Complex data requires specialized skills to ensure accuracy. Fields like medicine and science require domain experts so that appropriate information can be identified and labeled. Most of the time, data labeling work is outsourced to specialist image labeling companies with experience in labeling data in particular industry fields.
A proper approach, whether you want it done in-house, crowdsourced, or outsourced, will help get your work done better with access to specialized services. In-house is an option if you have your experts and infrastructure in place but may not be cost effective. Crowdsourcing will give you access to experts from across the globe to work on a particular task. Outsourcing is a great option where you hire domain experts for your labeling project. You have better control over your project as you build a temporary team that works as per your specifications providing technology-enabled solutions.
Conclusion
Data quality is the biggest challenge in data labeling. And there are associated challenges that annotators face regularly, like maintaining a specialist workforce, manual processes, finances, etc. Businesses need to take a more automated approach for quick and accurate labeling. With a fast leap in technology, processes, and evolution of systems in data annotation, things are getting more streamlined. Outsourcing is also an option to consider with various specialists offering high-quality specialized annotation services.
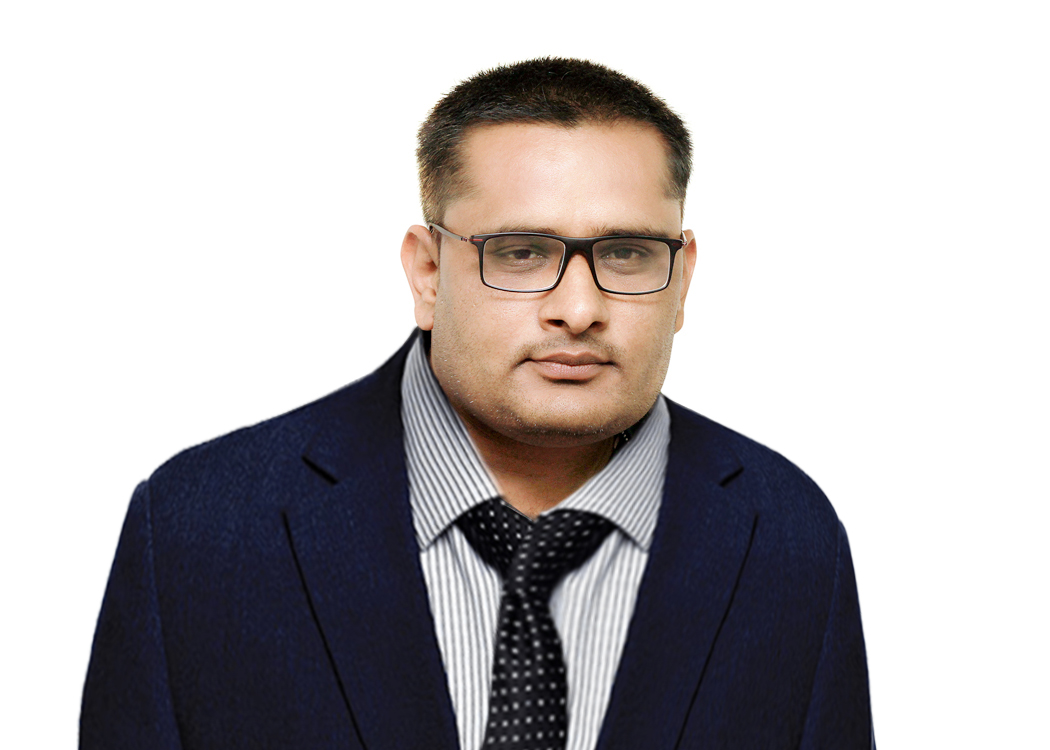
Snehal Joshi heads the business process management vertical at HabileData, the company offering quality data processing services to companies worldwide. He has successfully built, deployed and managed more than 40 data processing management, research and analysis and image intelligence solutions in the last 20 years. Snehal leverages innovation, smart tooling and digitalization across functions and domains to empower organizations to unlock the potential of their business data.